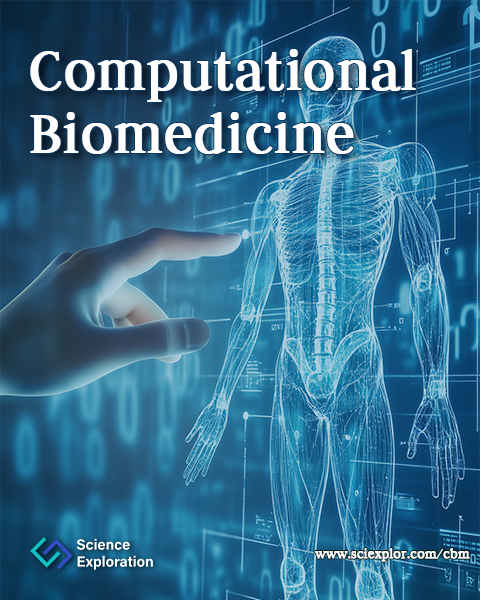
-
Computational Biomedicine is a peer-reviewed, open access journal published quarterly and owned by Science Exploration Press. The journal covers a wide range of topics, including molecular medicine, simulation, modeling techniques, imaging methods, and information technology. Our mission is to encourage scientists to publish their experimental and theoretical findings in a detailed open-access format. We invite submissions across various article types, including Research Articles, Review Articles, Editorials, Case Reports, Letters to the Editor, Perspectives, and Commentaries. more >
Articles
A comprehensive review on neuropeptides: databases and computational tools
-
Neuropeptides are crucial signaling molecules that regulate diverse physiological processes spanning growth, social behavior, learning, memory, metabolism, homeostasis, reproduction, and neural differentiation across both nervous and peripheral ...
MoreNeuropeptides are crucial signaling molecules that regulate diverse physiological processes spanning growth, social behavior, learning, memory, metabolism, homeostasis, reproduction, and neural differentiation across both nervous and peripheral systems. Dysregulation of neuropeptides signaling is closely linked to various pathological conditions, such as neurological disorders, metabolic diseases, cardiovascular conditions, and even cancer, positioning them as potential therapeutic agents or targets for intervention. In recent years, research into neuropeptides has accelerated, with vast amounts of data continuously accumulating in multiple databases. However, the study of neuropeptides is often impeded by the need for extensive and time-consuming experimental investigations. As a result, computational tools have become essential for the rapid, large-scale identification of neuropeptides. This review systematically discusses neuropeptide-related databases and computational tools. These databases organize extensive data on neuropeptide sequences, structures, and functions. Among these, NeuroPep2.0, with 11,417 neuropeptide entries, is currently the most widely used dataset for neuropeptide prediction. Additionally, this review explores the application of computational approaches in neuropeptide prediction. While early methods predominantly relied on homologous sequence alignment and biochemical feature statistics, recent advances in machine learning have significantly enhanced prediction accuracy and efficiency. Tools such as NeuroPred-PLM and DeepNeuropePred, developed by our research group using protein language models, have substantially improved prediction performance. In conclusion, this review provides a comprehensive overview of current neuropeptide databases and computational tools, offering researchers a thorough survey of available resources and analytical methods, and emphasizing the necessity of continuous optimization to advance neuropeptide research and its therapeutic applications.
Less -
Wei Xu, ... Yan Wang
-
DOI:https://doi.org/10.70401/cbm.2025.0001 - April 15, 2025
A comprehensive review on neuropeptides: databases and computational tools
-
Neuropeptides are crucial signaling molecules that regulate diverse physiological processes spanning growth, social behavior, learning, memory, metabolism, homeostasis, reproduction, and neural differentiation across both nervous and peripheral ...
MoreNeuropeptides are crucial signaling molecules that regulate diverse physiological processes spanning growth, social behavior, learning, memory, metabolism, homeostasis, reproduction, and neural differentiation across both nervous and peripheral systems. Dysregulation of neuropeptides signaling is closely linked to various pathological conditions, such as neurological disorders, metabolic diseases, cardiovascular conditions, and even cancer, positioning them as potential therapeutic agents or targets for intervention. In recent years, research into neuropeptides has accelerated, with vast amounts of data continuously accumulating in multiple databases. However, the study of neuropeptides is often impeded by the need for extensive and time-consuming experimental investigations. As a result, computational tools have become essential for the rapid, large-scale identification of neuropeptides. This review systematically discusses neuropeptide-related databases and computational tools. These databases organize extensive data on neuropeptide sequences, structures, and functions. Among these, NeuroPep2.0, with 11,417 neuropeptide entries, is currently the most widely used dataset for neuropeptide prediction. Additionally, this review explores the application of computational approaches in neuropeptide prediction. While early methods predominantly relied on homologous sequence alignment and biochemical feature statistics, recent advances in machine learning have significantly enhanced prediction accuracy and efficiency. Tools such as NeuroPred-PLM and DeepNeuropePred, developed by our research group using protein language models, have substantially improved prediction performance. In conclusion, this review provides a comprehensive overview of current neuropeptide databases and computational tools, offering researchers a thorough survey of available resources and analytical methods, and emphasizing the necessity of continuous optimization to advance neuropeptide research and its therapeutic applications.
Less -
Wei Xu, ... Yan Wang
-
DOI:https://doi.org/10.70401/cbm.2025.0001 - April 15, 2025
A comprehensive review on neuropeptides: databases and computational tools
-
Neuropeptides are crucial signaling molecules that regulate diverse physiological processes spanning growth, social behavior, learning, memory, metabolism, homeostasis, reproduction, and neural differentiation across both nervous and peripheral ...
MoreNeuropeptides are crucial signaling molecules that regulate diverse physiological processes spanning growth, social behavior, learning, memory, metabolism, homeostasis, reproduction, and neural differentiation across both nervous and peripheral systems. Dysregulation of neuropeptides signaling is closely linked to various pathological conditions, such as neurological disorders, metabolic diseases, cardiovascular conditions, and even cancer, positioning them as potential therapeutic agents or targets for intervention. In recent years, research into neuropeptides has accelerated, with vast amounts of data continuously accumulating in multiple databases. However, the study of neuropeptides is often impeded by the need for extensive and time-consuming experimental investigations. As a result, computational tools have become essential for the rapid, large-scale identification of neuropeptides. This review systematically discusses neuropeptide-related databases and computational tools. These databases organize extensive data on neuropeptide sequences, structures, and functions. Among these, NeuroPep2.0, with 11,417 neuropeptide entries, is currently the most widely used dataset for neuropeptide prediction. Additionally, this review explores the application of computational approaches in neuropeptide prediction. While early methods predominantly relied on homologous sequence alignment and biochemical feature statistics, recent advances in machine learning have significantly enhanced prediction accuracy and efficiency. Tools such as NeuroPred-PLM and DeepNeuropePred, developed by our research group using protein language models, have substantially improved prediction performance. In conclusion, this review provides a comprehensive overview of current neuropeptide databases and computational tools, offering researchers a thorough survey of available resources and analytical methods, and emphasizing the necessity of continuous optimization to advance neuropeptide research and its therapeutic applications.
Less -
Wei Xu, ... Yan Wang
-
DOI:https://doi.org/10.70401/cbm.2025.0001 - April 15, 2025